Larry Wasserman Elected to National Academy of Sciences
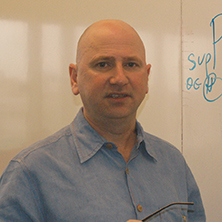
Acclaimed Carnegie Mellon University statistician Larry Wasserman has been elected to the National Academy of Sciences (NAS) in recognition of his distinguished and continuing achievements in original research. NAS membership is one of the highest honors a scientist can receive
Wasserman, a professor of statistics and machine learning who joined the CMU faculty in 1988, became a statistician because he enjoyed the combination of math and its applications. His research spans both theoretical and applied statistics. On the theoretical side, he focuses on the intersection of statistics and machine learning, which is becoming increasingly important in the era of big data as both work to analyze data for high-dimensional problems. His work has provided new methods and theory for simultaneously estimating the relationships between large numbers of variables and for finding subtle spatial structure in complex datasets.
"It's wonderful that the National Academy of Sciences has recognized Larry for his many scientific contributions to the field of statistics," said Tom Mitchell, E. Fredkin University Professor and former head of the School of Computer Science's Machine Learning Department. "Beyond those specific scientific contributions, Larry has played a key role guiding the evolution of statistical machine learning at CMU and beyond. In the late 1990s he was one of the first faculty to engage in a research center that evolved into CMU's Machine Learning Department."
Mitchell continued, "Larry has become one of our most beloved teachers, and his 2004 textbook 'All of Statistics' is popular worldwide."
"Larry's contributions to machine learning are too numerous to list," said Geoff Gordon, interim head of the Machine Learning Department, "but he has made fundamental contributions to research areas such as statistical topology, nonparametric inference and high-dimensional graphical models, as well as application areas such as astrophysics."