Welcome to our Virtual Graduate Student Orientation
We are extremely pleased that you have decided to become a member of our Department; we think it is a very special collection of faculty, staff and students. If you have any questions, please send an email to Diane Stidle.
Please join us remotely for our virtual graduate student orientation. Please visit CMU Zoom for information on how to use and download Zoom's client. For any questions and concerns, please do not hesitate to contact us.
Schedule
Friday, August 28, 2020
9:00 - 9:15 AM | Welcome to the Machine Learning Department | Roni Rosenfeld |
9:15 - 9:45 AM | Program Requirements: Separate talk for MS & PhD students | Katerina Fragkiadaki, Tom M. Mitchell |
9:45 - 10:00 AM | Break | |
10:00 - 10:15 AM | Welcome to the School of Computer Science | Martial Hebert |
SCS PhD Advisory Committee | Helen Zhou | |
10:15 - 10:30 AM | Deep learning: From an Alchemist to a theoretical Alchemist | Yuanzhi Li |
10:30 - 11:00 AM | Break | |
11:00 - 11:10 AM | Equilibrium models in deep learning | Zico Kolter |
11:15 - 11:25 AM | Diversity, Inclusion, and Impact efforts in MLD | Rayid Ghani, Zack Lipton, Leila Wehbe |
11:30 - 11:45 AM | Break | |
11:45 - 11:55 AM | Neural Architecture Search & Interpretability | Ameet Talwalkar |
12:00 - 12:10 PM | Privacy, Fairness, Algorithmic Economics | Steven Wu |
12:15 - 12:25 PM | Deep Learning / Reinforcement Learning | Russ Salakhutdinov |
12:25 - 1:00 PM | Break | |
1:00 - 1:10 PM | ML and Systems | Tianqi Chen |
1:15 - 1:25 PM | Large-scale ML: accuracy, efficiency, and fairness | Virginia Smith |
1:30 - 1:40 PM | Theory of unsupervised deep learning | Andrej Risteski |
1:45 - 1:55 PM | 3D neural visual perception, common sense learning | Katerina Fragkiadaki |
2:00 - 2:10 PM | Structured Prediction for NLP | Matt Gormley |
2:15 - 2:25 PM | RL for Autonomous Systems | Jeff Schneider |
2:25 - 3:00 PM | Break | |
3:00 - 3:10 PM | Forecasting Epidemics and Pandemics | Roni Rosenfeld |
3:15 - 3:25 PM | Image and video synthesis, deep generative model. | Jun-Yan Zhu |
3:30 - 3:40 PM | ML for Social Good, Fariness, Interpretability | Rayid Ghani |
3:45 - 3:55 PM | Deep Learning, Computer Vision and Reinforcement (Robot) Learning | Deepak Pathak |
3:55 - 4:15 PM | Break | |
4:15 - 4:25 PM | A Blueprint of Standardized and Composable Machine Learning: Theory, Algorithm, and System | Eric Xing |
4:30 - 4:40 PM | Assumption-free uncertainty quantification for ML | Aaditya Ramdas |
4:45 - 4:55 PM | Language in the brain and in machines | Leila Wehbe |
4:55 - 5:05 PM | ML for modeling virus infection pathways | Ziv Bar-Joseph |
5:10 - 5:20 PM | Beyond outcome fairness in the ML pipeline | Hoda Heidari |
Welcome to the School of Computer Science
A message from Martial Hebert, dean with the School of Computer Science at Carnegie Mellon University.
Virtual Livestream Recordings
Faculty Talks
Deep Learning: From an Alchemist to a Theoretical Alchemist by Yuanzhi Li
Currently accepting students? Yes, Master's and Ph.D.
Website | Contact
Equilibrium Models in Deep Learning by Zico Kolter
Currently accepting students? Yes, Master's and Ph.D.
Website | Contact
Toward the Jet Age of ML by Ameet Talwalkar
Currently accepting students? Yes, Master's and Ph.D.
Website | Contact
Machine Learning Meets Societal Values by Steven Wu
Currently accepting students? Yes, Master's and Ph.D.
Website | Contact
Machine Learning Systems by Tianqi Chen
In this presentation, Professor Chen talks about machine learning systems and a few essential elements to make a successful learning system. He then talks about potential research directions along the lines of machine learning compilers, learning to search structured space and scheduling in deep learning systems.
Currently accepting students? Yes, Master's and Ph.D.
Website | Contact
Large-scale ML: accuracy, efficiency, fairness by Virginia Smith
Currently accepting students? Yes, Master's and Ph.D.
Website | Contact
Theory of (mostly) unsupervised, (mostly) deep machine learning by Andrej Risteski
Embodied visual learning with neural 3D scene representations by Katerina Fragkiadaki
Machine Learning for Controlling Complex, Autonomous Systems by Jeff Schneider
Forecasting Epidemics by Roni Rosenfeld
Currently accepting students? Yes, Master's and Ph.D.
Website | Contact
Learning to Synthesize Images by Professor Jun-Yan Zhu
In this presentation, Professor Zhu dives into three research problems: (1) how machines can create realistic images, videos, and 3D data automatically (2) how machines can help humans create content more easily. (3) how humans can easily create and customize ML models.
Currently accepting students? Yes, Master's and Ph.D.
Website | Contact
ML/AI/Data Science for Social Good & Public Policy by Rayid Ghani
Currently accepting students? Yes, Master's and Ph.D.
Website | Contact
Towards Embodied Intelligence by Deepak Pathak
Currently accepting students? Yes, Master's and Ph.D.
Website | Contact
A Blueprint of Standardized and Composable ML: Theory, Algorithm, and System by Eric Xing
Currently accepting students? Yes, Master's and Ph.D.
Website | Contact
Assumption-free uncertainty quantification for ML by Aaditya Ramdas
Language in the brain and machines by Leila Wehbe
Machine learning for single cell analysis by Ziv Bar-Joseph
Beyond Outcome Fairness in the ML Pipeline by Hoda Heidari
Currently accepting students? Yes, Master's and Ph.D.
Website | Contact
Next-Gen Statistical Machine Learning by Pradeep Ravikumar
In this presentation, Professor Ravikumar talks about how next-gen statistical machine learning is flexible, graceful and transferable.
Graduate Student Assembly
The Graduate Student Assembly (GSA) is the student government branch that represents all graduate students at Carnegie Mellon. Funding for the GSA comes from graduate students' student activities fees.
Primary functions
- Organizing social events throughout the year
- Advocating on issues important to graduate students
- Providing funding for graduate organizations and professional development
For orientation information regarding the Graduate Student Assembly, please visit their slide-deck.
Career and Professional Development Services
For Incoming PhD Students
For Incoming MS Students
Women@SCS
Women@SCS directs TechNights (Creative Technology Nights), aiming to expand the diversity of interest in computing among middle school students. Using computer animation, web design, programming, robotics, and interactive media, we hope to engage a more diverse generation of future technologists.
If you'd like to volunteer with TechNights, please contact Olivia (Liv) Zane (ocz@andrew.cmu.edu) to be added to our email list and stop by any Monday night! Volunteers are always welcome, and you don't need to commit in advance or have prior experience with the topics. If you'd like to get a little more involved and lead a session, please come to our planning meeting at the start of each semester to pitch your ideas, and/or contact Natalie Sauerwald (nsauerwald@cmu.edu).
SCS PhD Advisory Committee
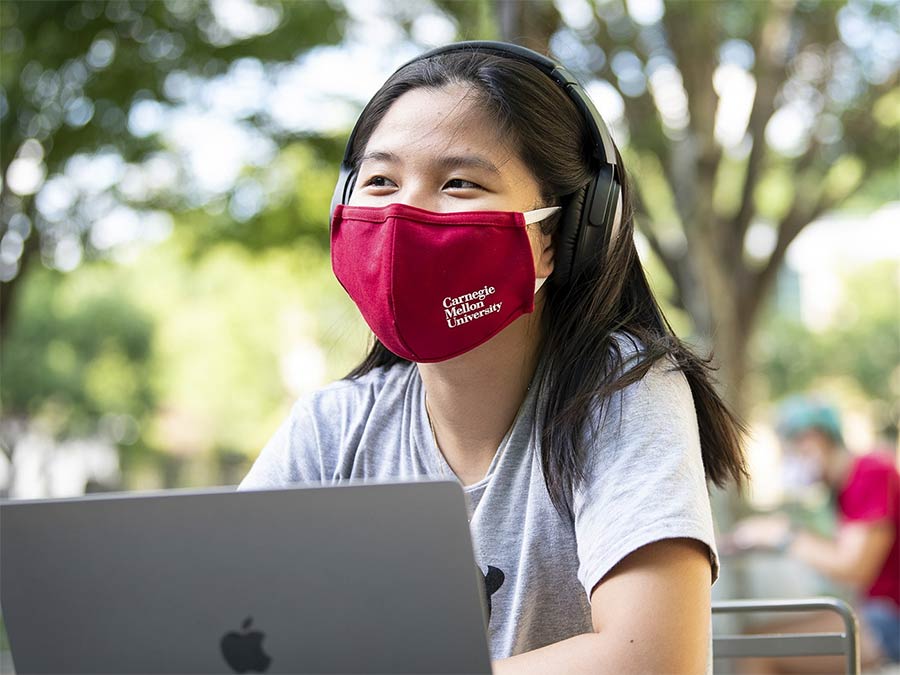
The moment has finally arrived. You’re about to embark upon your Ph.D. journey at CMU. The road in front of you is long, but you aren’t alone. You’re now a part of the SCS community.
Below are some resources we have assembled to help start your Ph.D. journey from all of us with the SCS Ph.D. Advisory Committee.
- Please view our committee’s welcome presentation for Fall 2020 on Google Drive.
- Welcome to SCS Website.
- Also, please visit the SCS Doctoral Student Review: A Student Perspective.
Resources
The Machine Learning Department has a vast variety of phenomenal research reading groups. For a full list of reading groups by research area, please check out this Google Spreadsheet.
CMU Libraries: Please visit this presentation for an introduction on how to use CMU Libraries.
SCS Computing Guidance
This video offers an overview of the SCS computing environment for new users at the School of Computer Science.
Please know that this guide does not intend to be a comprehensive set of instructions, but an excellent place to start gaining familiarity with our computing environment.
Through the video guide, there are links to further information about SCS Computing Facilities.
Graduate Entrepreneurship
Membership Benefits
- Opportunities to collaborate with graduate students on startup projects.
- Guided Introduction to CMU's entrepreneurial ecosystem.
- Assistance with recruiting for or founding a startup.
- Connections to founders, investors, and mentors from Pittsburgh and beyond.
The GEC fosters opportunities for all graduate students at CMU to develop and exercise their entrepreneurial thinking. Through GEC's founders and investors' network, they will help you with the skills you need to succeed as an entrepreneur.
Learn more and obtain further details by visiting the Graduate Entrepreneurship Club.
