CDC Says Delphi Research Group's Flu Forecasts Once Again Most Accurate
Delphi Research Group Epidemiological Forecasting Systems Get Top Marks Four Years in a Row
The U.S. Centers for Disease Control and Prevention has announced that Carnegie Mellon University's forecasts of national and regional influenza activity during the 2017-2018 flu season were the most accurate of the 30 systems in its flu forecasting initiative.
Carnegie Mellon's Delphi Research Group has proven the most accurate four years in a row and for four of the five years that the CDC has run the forecasting initiative. Last season, 21 research groups participated, testing 30 different forecasting systems.
"The CDC seems very happy with this entire initiative," said Roni Rosenfeld, Delphi leader and head of the Machine Learning Department. "Flu forecasts were actually used in official CDC communications for the first time in the 2017-2018 season."
Delphi fielded two systems. The first uses machine learning to make predictions based on both past patterns and input from the CDC's domestic flu surveillance system. The second system bases its predictions on the judgments of human volunteers who submit their own weekly predictions — the so-called wisdom-of-crowds approach.
CMU is using updated versions of both systems to forecast the current season, which thus far is off to a mercifully slow start. The forecasts are available through the Delphi website, and the group welcomes input from anyone who wants to contribute to the weekly crowdsourced forecasts.
The flu forecasts by CMU and other participants suggest flu activity will rise in the next few weeks, but Rosenfeld said it's too soon to say when flu activity will peak or how high it might get.
Unlike flu surveillance, which tracks flu activity based on reports of flu-like illnesses from physicians, flu forecasting attempts to look into the future, much like a weather forecast, so health officials can plan ahead. Delphi and the other forecasting groups make weekly predictions throughout the flu season. The CDC combines those efforts in its FluSight Network, with forecasts available online.
In addition to CMU's Delphi team, the CDC's forecasting initiative includes groups from entities such as Columbia University, Los Alamos National Laboratory and the University of Massachusetts-Amherst.
Initially, the CDC asked participants to forecast flu-related visits to physician offices in each of 10 regions in the nation. But last year, the CDC added two additional forecasting challenges — flu-related visits to physician offices for each state and flu-related hospitalization nationwide for each of five age groups.
Delphi co-leader Ryan Tibshirani, associate professor of statistics and machine learning, said forecasting by state is important because flu activity can be very local, so wide differences are possible even within a region. Forecasting hospitalization is important, he added, because some strains of flu can result in more severe illness than others, so flu activity levels in hospitals can differ markedly from activity levels in doctors' offices.
CMU participated in all three challenges, submitting the most accurate forecasts for each one. The machine learning method proved most accurate for the state-by-state and hospitalization forecasts, while the wisdom-of-crowds method was most accurate for regional forecasts.
"Virtually all members of the Delphi group contributed to our success, with theory, algorithmic development, implementation and weekly participation in the crowdsourcing system," Rosenfeld said. "Special kudos to the students who oversaw the competition weekly, if not daily, during the unusually long flu season." Those students included Logan Brooks, a Ph.D. student in the Computer Science Department; Aaron Rumack, a Ph.D. student in machine learning; and Jiaxian Sheng, a senior computer science major.
The Delphi group unites students and faculty from the Machine Learning, Statistics and Data Science, Computer Science and Computational Biology departments. The forecasting efforts are supported by the Machine Learning for Good fund established at the School of Computer Science by Uptake, the Defense Threat Reduction Agency, and the National Institute of General Medical Sciences' Models of Infectious Disease Agency Study (MIDAS).
CDC Says Delphi Research Group's Flu Forecasts Once Again Most Accurate

By Byron Spice
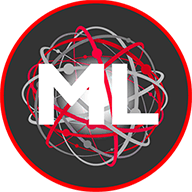