Towards the Jet Age of Machine Learning

Machine Learning today resembles the dawn of aviation. In 1903, dramatic flights by the Wright brothers ushered in the Pioneer Age of aviation, and within a decade, there was widespread belief that powered flight would revolutionize transportation and society more generally. Machine Learning today is also rapidly advancing. We have recently witnessed remarkable breakthroughs on important problems including image recognition, speech translation, and natural language processing, and major technology companies are investing billions of dollars to transform themselves into ML-centric organizations. There is a growing conviction that Machine Learning holds the key to some of society’s most pressing problems.
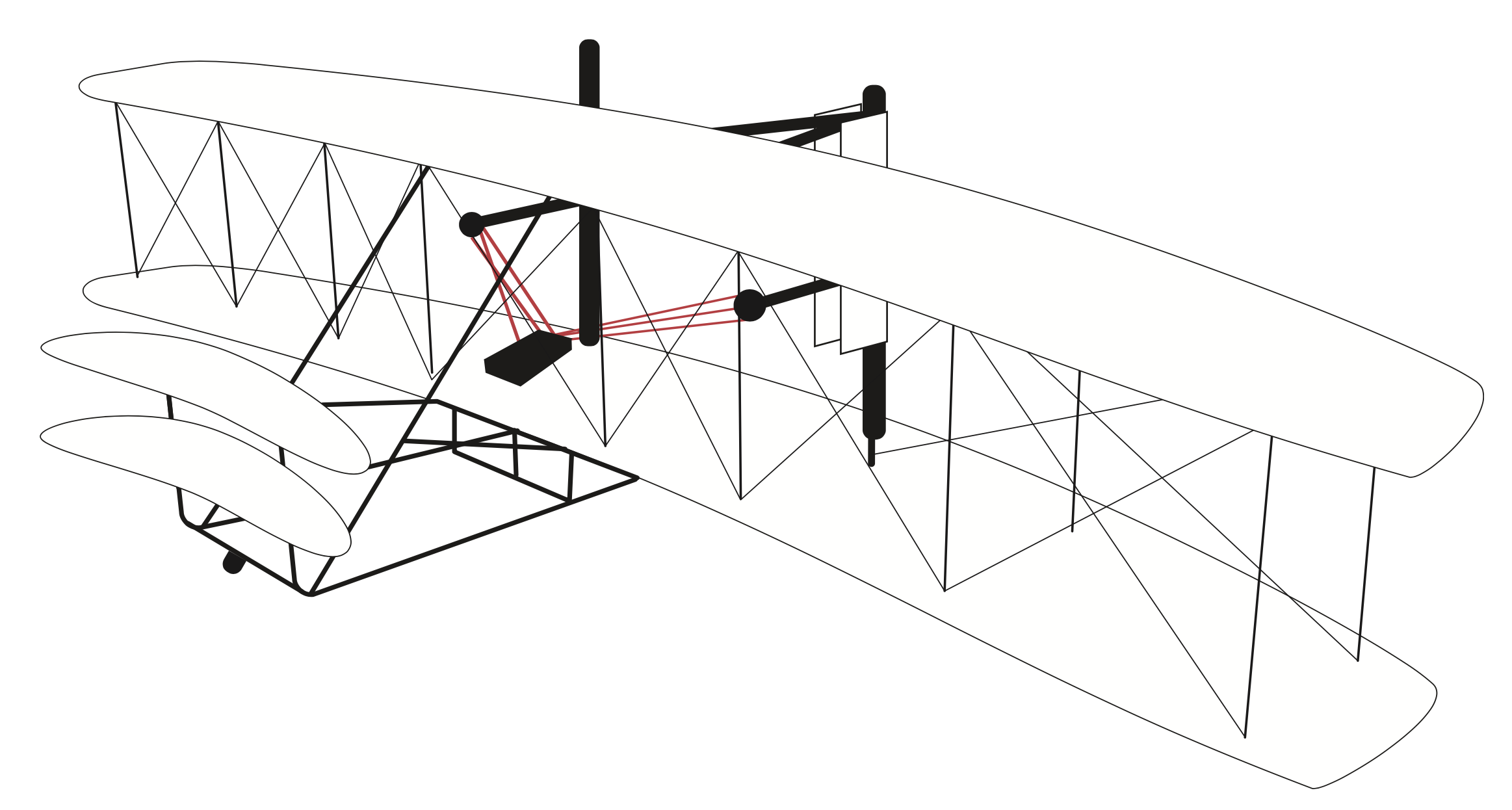
However, this excitement should also be met with caution. For all the enthusiasm that the Wright brothers generated, nearly half a century would pass before widespread commercial aviation finally became a reality. During the Pioneer Age, aviation was largely restricted to private, sport, and military use. Getting to the Jet Age required a series of fundamental innovations in aeronautical engineering—monoplane wings, aluminum designs, turbine engines, stress testing, jumbo jets, etc.
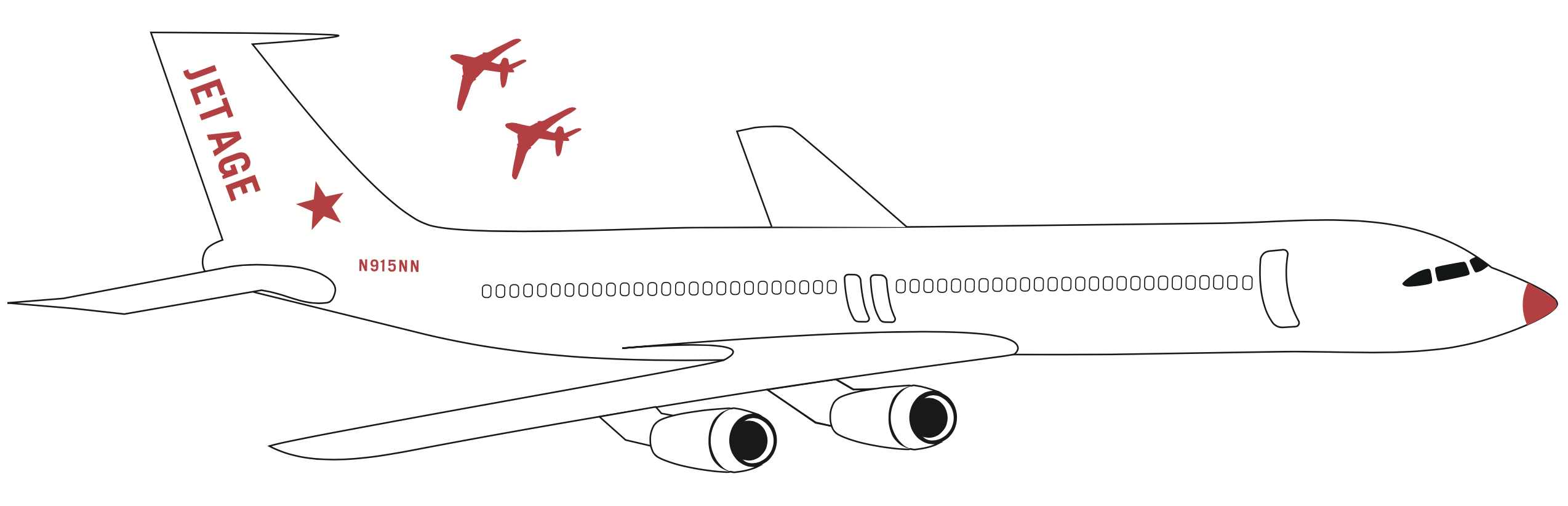
Simply put, we needed to invent aeronautical engineering before we could transform the aviation industry. Similarly, we need to invent a new kind of engineering to build Machine Learning applications. Data-driven software development is radically different from conventional software development, as it targets complex applications domains (e.g., vision, speech, language) and focuses on learned behaviors instead of rule-based operations (e.g., training deep neural networks on massive data sets versus hand-coded if-then-else statements). Currently, very few organizations have the expertise to do this kind of engineering, and we are just scratching the surface of the potential for ML-powered technology. We describe three key challenges of this new development paradigm below.
So what are the challenges that we are facing when implementing modern Machine Learning applications on the aviation industry?
For a full read please visit: https://goo.gl/cbWXVM
Article, courtesy of Dr. Ameet Talwalkar – O’Reilly Media, Inc.