Kass Co-Authors 10 Simple Rules to Use Statistics Effectively
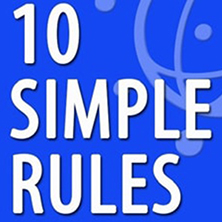
Under growing pressure to report accurate findings as they interpret increasingly larger amounts of data, researchers are finding it more important than ever to follow sound statistical practices.
For that reason, a team of statisticians including Carnegie Mellon University’s Robert E. Kass wrote “Ten Simple Rules for Effective Statistical Practice.” Published in PLOS Computational Biology for the journal’s popular “Ten Simple Rules” series, the guidelines are designed to help the research community — particularly scientists who aren’t statistical experts or without a dedicated statistician as part of their team — understand how to avoid the pitfalls of well-intended, but inaccurate statistical reasoning.
“A central and common task for us as research investigators is to decipher what data are able to say about the problems we are trying to solve,” wrote Kass, professor of statistics and machine learning and interim co-director of the Center for the Neural Basis of Cognition, and his co-authors. “Statistics is a language constructed to assist this process, with probability as its grammar.”
A summary of the 10 rules:
#1 – Statistical Methods Should Enable Data to Answer Scientific Questions
Collaborating with statisticians is often most helpful early in an investigation because inexperienced users of statistics often focus on which technique to use to analyze data, rather than considering all of the ways the data may answer the underlying scientific question.
#2 – Signals Always Come With Noise
Variability comes in many forms, but it is crucial to understand when it is good and when it is noise in order to express uncertainty. It also helps to identify likely sources of systematic error.
#3 – Plan Ahead, Really Ahead
Asking questions at the design stage can save headaches at the analysis stage. Careful data collection also can greatly simplify analysis and make it more rigorous.
#4 – Worry About Data Quality
When it comes to data analysis, “garbage in produces garbage out.” The complexity of modern data collection requires many assumptions about the function of technology, often including data pre-processing technology, which can have profound effects that can easily go unnoticed.
#5 – Statistical Analysis Is More Than a Set of Computations
Statistical software provides tools to assist analysis, not define them. The scientific context is critical, and the key to principled statistical analysis is to bring analytical methods into close correspondence with scientific questions.
#6 – Keep it Simple
Simplicity trumps complexity. Large numbers of measurements, interactions among explanatory variables, nonlinear mechanisms of action, missing data, confounding, sampling biases and other factors can require an increase in model complexity. But, keep in mind that a good design, implemented well, can often allow simple methods of analysis to produce strong results.
#7 – Provide Assessments of Variability
A basic purpose of statistical analysis is to help assess uncertainty, often in the form of a standard error or confidence interval, and one of the great successes of statistical modeling and inference is that it can provide estimates of standard errors from the same data that produce estimates of the quantity of interest. When reporting results, it is essential to supply some notion of statistical uncertainty.
#8 – Check Your Assumptions
Widely available statistical software makes it easy to perform analyses without careful attention to inherent assumptions, and this risks inaccurate, or even misleading, results. It is therefore important to understand the assumptions embodied in the methods and to do whatever possible to understand and assess those assumptions.
#9 – When Possible, Replicate!
Ideally, replication is performed by an independent investigator. The scientific results that stand the test of time are those that get confirmed across a variety of different, but closely related, situations. In many contexts, complete replication is very difficult or impossible, as in large-scale experiments such as multi-center clinical trials. In those cases, a minimum standard would be to follow Rule 10.
#10 – Make Your Analysis Reproducible
Given the same set of data, together with a complete description of the analysis, it should be possible to reproduce the tables, figures and statistical inferences. Dramatically improve the ability to reproduce findings by being very systematic about the steps in the analysis, by sharing the data and code used to produce the results and by following accepted statistics best practices.
In addition to Kass, the co-authors are Johns Hopkins University’s Brian S. Caffo, North Caroline State University’s Marie Davidian, Harvard University’s Xiao-Li Meng, Bin Yu of the University of California Berkeley, and Nancy Reid of the University of Toronto.
“I am a big believer in the value of identifying major ideas in statistics, and stating them clearly and concisely,” Kass said. “The 10 simple rules series is terrific, having proven its worth as a format for high-level scientific concepts. This article was pretty hard work, but we had a great team and I was extremely happy with the result.”